Integrating AI in 2024: best LLM use cases for startups
Step by step we are moving to 2024 and this year should be definitely promising in terms of new AGI and Generative AI opportunities.
According to Forbes, the next generation of Generative AI stands as a top-5 trend in 2024, competing with Augmented Working, Ethical AI, AI Legislation, and Quantum AI. So, 2024 sounds really promising in terms of new ways of artificial intelligence implementation, especially for companies looking to implement GPT, Bard, or other closed-source large language models.
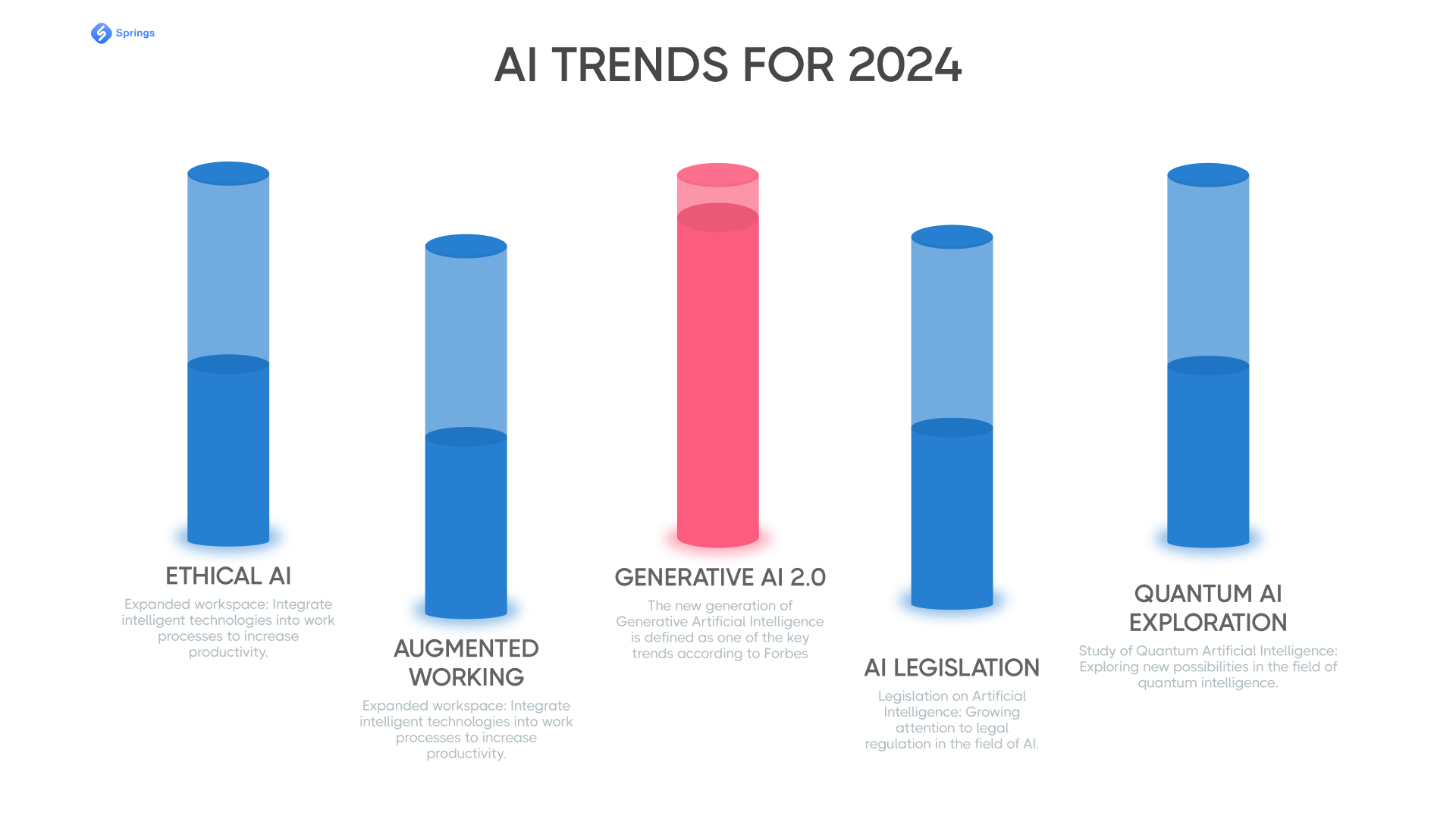
OpenAI GPT Use Cases
OpenAI’s GPT as the main large language model leader in the GenAI industry has already proved its power with GPT 3 implementation to many different software solutions. Its follower GPT-3.5 which was initially used in well-known ChatGPT made a significant boost in large language modeling.
We may see hundreds or even thousands of new ChatGPT-based startups that were launched in 2023. It seems to be like a new Startup OpenAI trend that filled the AI startup market with different interesting solutions, starting from integrating ChatGPT to Chrome Extension and finishing with an ongoing GPT-4 fine-tuning models integrated into LMS.
The field for the LLM use case is unbordered. GPT-3.5 API and GPT-4 API can be integrated into traveling businesses, real estate, hospitality, eCommerce, customer support chatbots, and many other domains.
The other great example of ChatGPT API integration is sales and marketing SaaS. A lot of startups in this field have already implemented ChatGPT into their existing solutions and we may see tons of new companies implementing AI day by day.
According to Statista, the top 10 industries that are using ChatGPT in their businesses are Technical, Education, Business Services, Manufacturing, Finance, Retail, Health Care, Government, Media and Construction. And seems like 2024 will be pretty much the same in this way.
OpenAI’s CEO Sam Altman has already announced the development of GPT-5, and considering they allocated Microsoft as the main partner for this path, - that should be something superintelligent and obviously more human-like (Tom’s Guide). So, it can be absolutely new route for other industries to join AI ventures.
Open-Source LLM Startups
Have you ever thought that well-known ChatGPT, Bard, and Cohere are closed-source large language model examples? It means that they can be used only within those coded functionality that is provided by their owner and can’t be fully modified by our development team and specific needs.
However, more and more open-sourcе LLMs can be used as a great alternative to closed ones with their benefits for startups and businesses. Let’s dive deeper into this and see how startups use open source large language model.
According to Finxter, the most used open-source LLMs in 2023 were:
- OPT with 180 billion tokens
- BLOOM with 341 billion tokens
- LLaMa with 1.4 trillion tokens
- MPT with 1 trillion tokens
- Falcon with 1.5 trillion tokens
- LLaMA 2 with 2 trillion tokens
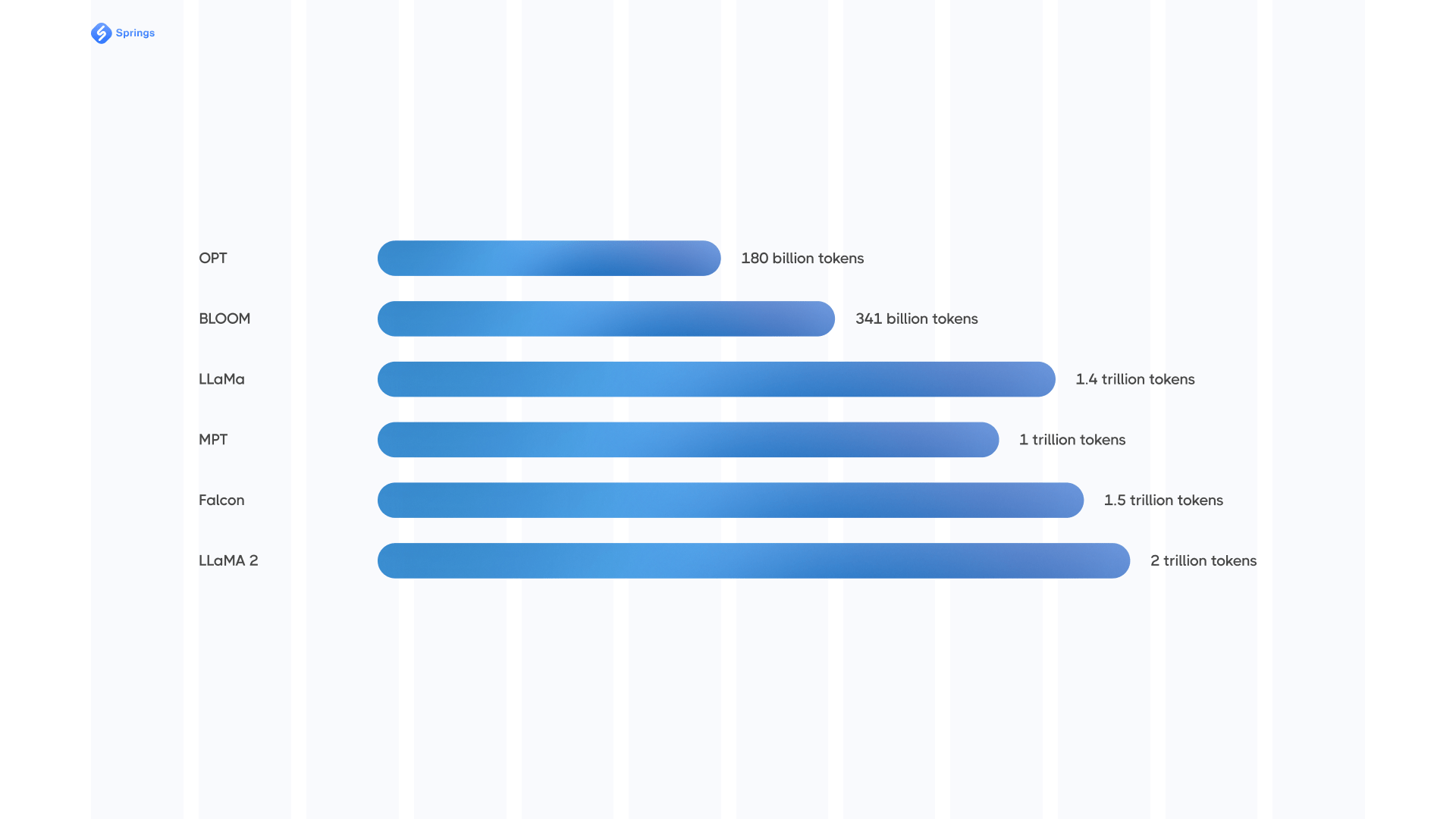
One of the biggest advantages of open source large language models is that they can be much faster in terms of execution time. For instance, closed-source ChatGPT sometimes needs up to 13 seconds to proceed query while such open-source model as LLM LaMini needs up to 5 seconds to proceed the same one.
Open source LLMs are used in different industries but they are really useful, especially in software engineering-oriented startups and this trend will obviously grow in 2024. A great example of such a fast-growing company is Hugging Face. Their platform has over 25000 models, 40000 datasets, and 50000 demo apps (Spaces). All of them are open source and publicly available, so customers can easily collaborate and build ML and AI applications.
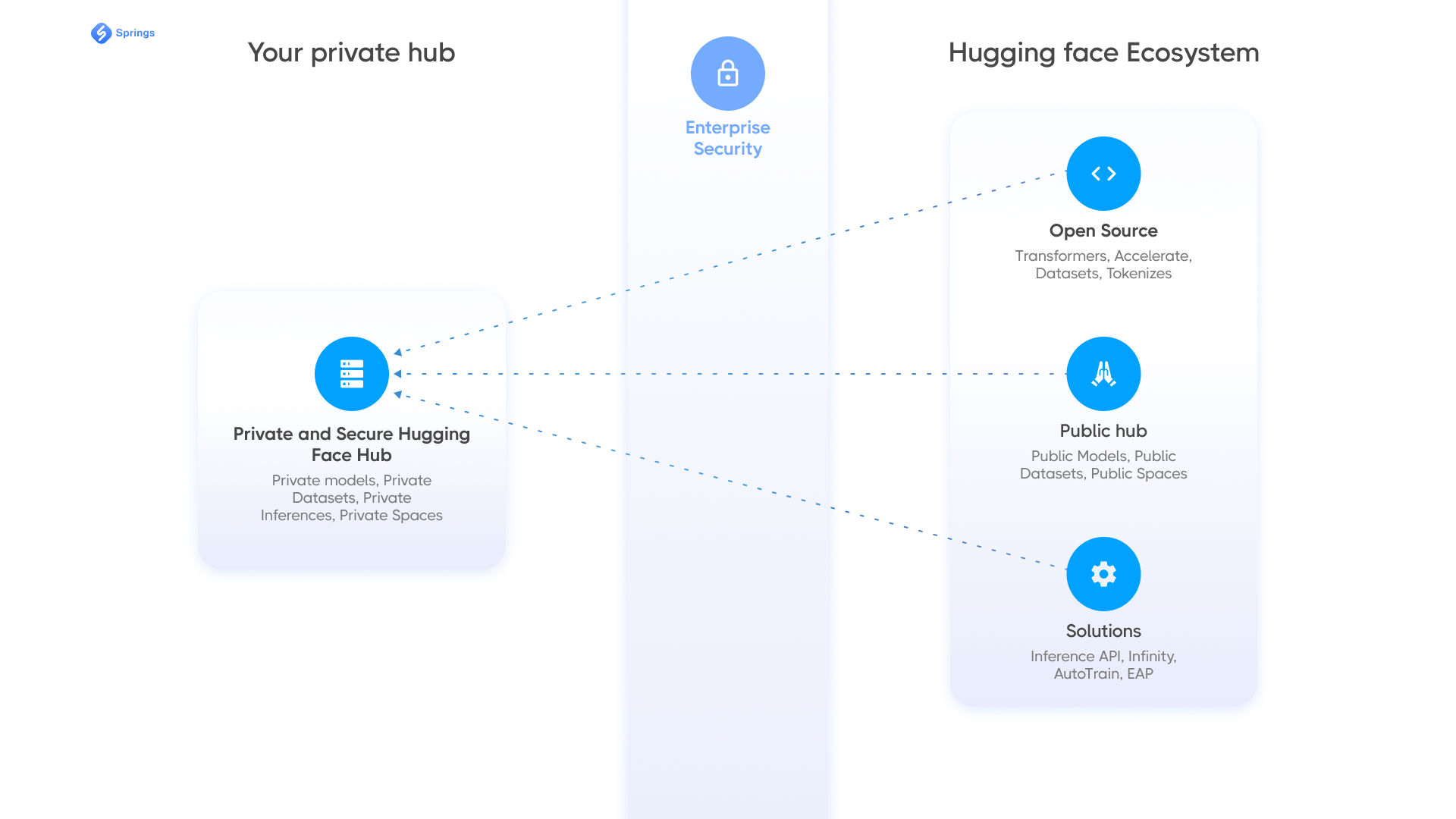
Another type of open source large language model use case could be its integration into existing software where closed-source LLM can’t provide the necessary results. For example, imagine you want your customer support AI Chatbot to answer 2x faster to the incoming inquiries, but current closed-source model like Bard or GPT-3.5 can’t provide the needed speed. In this case, you may look at open-source models like LLaMA 2, Falcon, or Flan-T5.
So, obviously open source large language model use case could be a great opportunity for your new project idea or startup, especially if you are aiming to create some state-of-art solution in machine learning or artificial intelligence fields.
Solutions with Сustom Language Models
Implement GPT and other types of prompt engineering has already become a trend. But language models are not always pre-defined and they could be customized. What is a custom language model and what could be custom large language model examples? Let’s have a look.
The Large Language Model is a pre-trained machine learning model designed to perform a variety of tasks: from sentiment analysis to language translation. At the same time, the Custom Language Model is already a fine-tuned model or trained specifically for a certain domain, use case or industry. The Custom Language Model can be used to meet the unique needs of a business or use case.
The specifics of a custom language model lie in fine-tuning. According to OpenAI API fine-tuning provides you with:
- Possibility to train the model on more examples than can fit in a prompt
- Better quality results than just prompting
- Token savings due to shorter prompts
For example, OpenAI's text generation models have been pre-trained on a vast amount of text. Their fine-tuning improves on few-shot learning by training on many more examples than can fit in the prompt, letting you achieve better results on a wide number of tasks. As soon as a model has been fine-tuned, you won't need to provide as many examples in the prompt. This saves costs and enables lower-latency requests.
So, in total, we may diversify custom language models and large language models in the following way:
In general, custom LLM is like a decentralized version of a basic large language model. Let’s see how it can be implemented in different software solutions. According to Garper, almost 175 Zettabytes of data will be present at the end of 2025 which means that businesses need to spend efforts on analyzing their data and finding out trends and patterns.
A lot of startups have witnessed growth as a result of implementing custom LLMs into their businesses. The industries to integrate custom llm use case can be different:
1. Informational Technology
IT and software startups will be the most popular users of custom LLMs in 2024. Gpt 3 implementation and its further fine-tuning has already become a popular trend among SaaS, PaaS, or IaaS startups in 2023 and their number are growing significantly day by day. There are many effective ways to implement LLM in IT industry depending on your business needs, here are some IT llm use cases:
- Cybersecurity: threat detection, anomaly analysis, and generating security-related documentation.
- Product Management: generating status reports, providing insights into project data, assisting in task prioritization, etc.
- Data monitoring: interpretation of logs, alerts, and other data for more effective and proactive system management.
- Autotesting: AI generation of test cases, test scripts, and analyzing of test results.
2.Sales & Marketing
Custom language models can streamline the process of generating content for blogs, product descriptions, social media posts, and marketing campaigns, enabling organizations to conserve time and resources. This, in turn, empowers individuals to concentrate on vital tasks such as sales and revenue generation. There are many options in integration custom language models to sales or marketing startups, for exmaple:
- Reviews: analyzing customers reviews and reply to them immediately basing on the prompts or policy of your company.
- Sales Calls: providing real-time suggestions during sales calls, helping tailor sales pitches to individual customer needs.
- Lead Generation: analyzing online conversations and interactions while qualifying leads based on predefined criteria.
- SEO: generating keyword-rich content, meta tags, and optimizing website copy for better search engine visibility.
- Market Research: analyzing market trends, customer feedback, and competitor activities, providing valuable insights for strategic decision-making.
- Email Marketing: generating personalized email content based on your ICP and UVP preferences.
3. Customer Support & Experience
In the field of customer support and experience, AI Chatbots and AI Assistants based on custom LLMs have already started to replace human agents. Customized AI Chatbots are extensively employed to address customer queries, resolve issues, and provide guidance throughout various processes. Meanwhile, AI-based Assistants aid employees in tasks like scheduling meetings and managing calendars. Both forms of digital assistance contribute significantly to enhancing overall customer support. Customer Experience is a huge field for LLM implementation and customizing, let's have a look at some examples:
- 24/7 Help Desk: with integration of LLM-based chatbot you can be sure all your customer inquires are non-stop processed.
- AI-powered CXM: automating customer experience management systems by understanding customer support tickets, directing them to the appropriate department, and providing initial responses.
- Multilingual Support: responding to customer queries in multiple languages, ensuring a more inclusive and accessible customer support experience.
- Interactive FAQ: creating of interactive FAQs that dynamically adapt based on customer queries, offering a more engaging and effective way for users to find information.
- Call to Action: state-of-art LLMs are able to perform any functions, such as engaging customer to book a call or provide email when necessary.
- Conversation Analysis: addressing potential issues proactively and improving overall customer satisfaction by analyzing customer interactions and conversations.
4. Big Data
Big Data analysis and processing is a huge industry to implement ML models. Custom large language models have the capacity to examine user-generated data, such as reviews or feedback, extracting valuable insights through sentiment analysis. Moreover, they may rebuild the data in different types and pieces using vector databases.
- Reports Generation: custom LLM implementation helps in the creation of comprehensive and insightful reports without the need for manual report writing.
- Compliance: ensuring compliance with regulatory standards and identifying potential security threats
- Automated ETL Processes: LLMs help in Extract, Transform, Load (ETL) processes to automate data integration tasks, map transformations, and generate code snippets for data processing pipelines.
- Time-Series Analysis: helping data scientists generate code snippets for tasks such as trend analysis, anomaly detection, and forecasting.
5. Healthcare
Such types of LLMs play a crucial role in different medical diagnoses by analyzing patient data and predicting appropriate measures based on symptoms. Additionally, specified customized language models assist healthcare professionals in medical transcriptions, simplifying the documentation and data process. Custom language models can bring benefits while their integration in healthcare industry:
- Patient Monitoring: analyzing data from remote IoT devices, helping healthcare professionals track different patient health metrics.
- Diagnostic: LLMs help in analyzing medical imaging reports, lab results, and patient histories to aid in diagnosis and treatment planning.
- Appointments: automation of appointment scheduling, sending reminders, and improving communication between healthcare providers and patients.
- Medical Transcription: converting spoken words into written text, and improving the accuracy and efficiency of medical documentation.
- Patient Education: AI LLM integration into medical documentation and software helps patients receive easily understandable information about their conditions, treatments, and medications.
6. HR & Recruitment
Custom LLMs and other types of ML models can be a great benefit for HR automation and recruitment processes with artificial intelligence implementation. The HR companies implementing AI recruitment solutions with custom LLMs have already shown impressive ROI results in 2023 and 2024 should be even more productive as the AI algorithms are being improved day by day. Here are some examples of how LLMs may benefit HR and Recruitment processes:
- Performance Assistance: assisting employees during the performance review process by generating suggested feedback, improvement plans, and development goals.
- Feedback Analysis: analyzing employee feedback from surveys and performance reviews, extracting insights to inform talent development strategies and improve employee engagement.
- Interview Scheduling: LLMs help in automated interview scheduling, considering the availability of both candidates and interviewers to streamline the process.
- Candidate Sourcing: help in candidate sourcing by analyzing online profiles, social media, and professional networks to identify potential candidates.
- CV Screening: automated resume screening, allowing the system to analyze resumes and match candidates with job requirements, streamlining the initial stages of recruitment.
The future belongs to AI and ML companies. According to the scheduled product launches on Product Hunt in early 2024, we will expect tons of new AI startups.
We may notice that almost 25% from the startups are related to different AI-powered solutions, and some of them will be definitely using Large Language Models examples that we discussed today: starting from GPT-4 integration or fine-tuned LLaMa models, AI text-to-video generation tools and creating the design using third-parties AI integrations like MidJourney or Stabble Diffusion.
LLM use case is obviously something that technical startups will be looking for in 2024. Smart text generation is a progressive step into business automation and saving costs on human resources, so more and more investors are eager to allocate millions of dollars to the solutions or even ideas that could bring great ROIs in a year or two.